How to Avoid Common Mistakes in A/B Testing
When it comes to improving your website or app, there’s one classic method that digital marketers swear by: A/B testing. Also known as split testing, this method involves comparing two versions of the same webpage to see which one performs better. It’s a great way to make changes to your website while being confident they’ll actually improve user experience or conversion rates.
But here’s the kicker: A/B testing, when not done right, can lead to inaccurate conclusions and sub-optimal decisions. And worst of all, you might not even realize you’re doing it wrong. That’s why we’re going to help you avoid these common mistakes.
Mistake 1: Testing Too Many Elements at Once
A common error while conducting A/B tests is trying to test too many variations at once. Although multi-variate tests have their place and can be useful in certain scenarios, overcomplicating your A/B tests can lead to confusing and inconclusive results.
Picture this: you're testing two versions of a landing page – version A is the original, and version B is the alternative. In the alternative version, you've made a slew of changes – the headline, the main image, the button colour, and the page layout.

After running your test, you find that version B outperforms version A. You're glad, and novel design B becomes the new default. But an important question arises: what led to the improvement? Was it the revised headline, the new image, the button colour change or did the new layout work wonders?
Unfortunately, as you've made several changes in version B, it's tough to pin down what exactly caused the performance uptick. This situation is what testers refer to as "interaction effects", where multiple alterations can interact and produce unpredictable effects.
This problem of 'testing too many elements at once' often leaves you without clear direction for future testing or a definitive understanding of what your users prefer. The results become muddied, and the valuable insights you hoped to gain from the experiment become less actionable.
To avoid this common pitfall, adopt a more restrained and systematic approach—test one variable at a time. This method is known as "Isolated Testing" or "One Variable Testing", and it involves changing one element for each test while other elements remain constant.
For instance, if you want to improve the conversion rate of a sign-up form, start by tweaking one element—say, the form headline—while keeping the rest of the form unchanged. If the adjusted form outperforms the original form, you can attribute the improvement in performance to the new headline confidently.
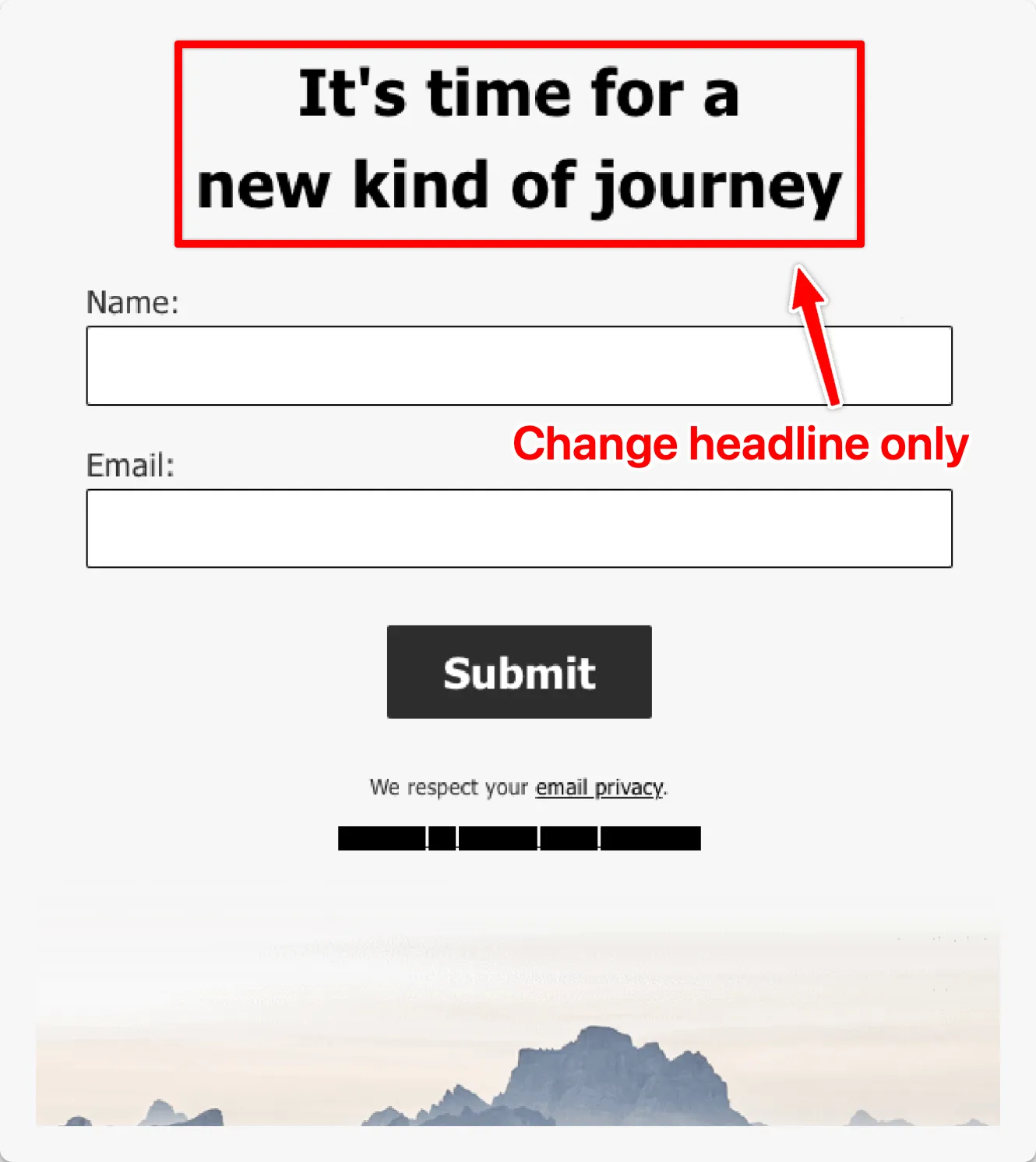
Isolated testing allows marketers to hone in on a precise understanding of how changing individual elements of a webpage or marketing piece impacts its performance. So even though testing this way may seem slower, it actually gives you clearer, more actionable insights.
Mistake 2: Not Giving Your Test Enough Time
Anyone pressing the speed pedal in A/B testing should be aware of how it relates to the concept of statistical power. Rushing to conclude your test can lead to what’s called ‘false positives’. These can give you the illusion that one version is better when the reality is that you simply didn’t run the test long enough for the results to stabilize.
Experts typically recommend running the A/B test for at least a week or two. Running your test for this duration ensures that you have enough data to make statistically reliable conclusions. This is because the audience behaviour tends to average out over the week, and any day-specific anomalies are evened out by other operating days.
Now, this brings us to the concept of ‘sample size’. The size of your sample plays a vital role in obtaining actionable A/B test results. A common mistake testers often make is not understanding the impact of a small sample size on the accuracy and reliability of the test results.
The good news is, you can eliminate this issue by using a Sample Size Calculator. This calculator uses three primary parameters for attaining the optimal sample size:
- Base Conversion Rate (CR%): This is your control group’s conversion rate before making any changes. Knowing your base rate helps you understand where you’re starting from and gauges any uplift in the results.
- Minimum Detectable Effect (MDE): This defines the smallest change that you care about detecting. It’s essential to remember that a smaller MDE would require a larger sample size. For instance, if you want to detect a change as small as ±5% from your base conversion, you’ll need a larger sample size than if you were aiming for a ±20% change.
- Statistical Significance: This is the likelihood that the difference in conversion rates between A and B is not due to random chance. In simpler words, it reflects the tester’s confidence in the outcome of the test. A common value used in A/B testing is 95%, implying there’s only a 5% probability that the results occurred randomly.
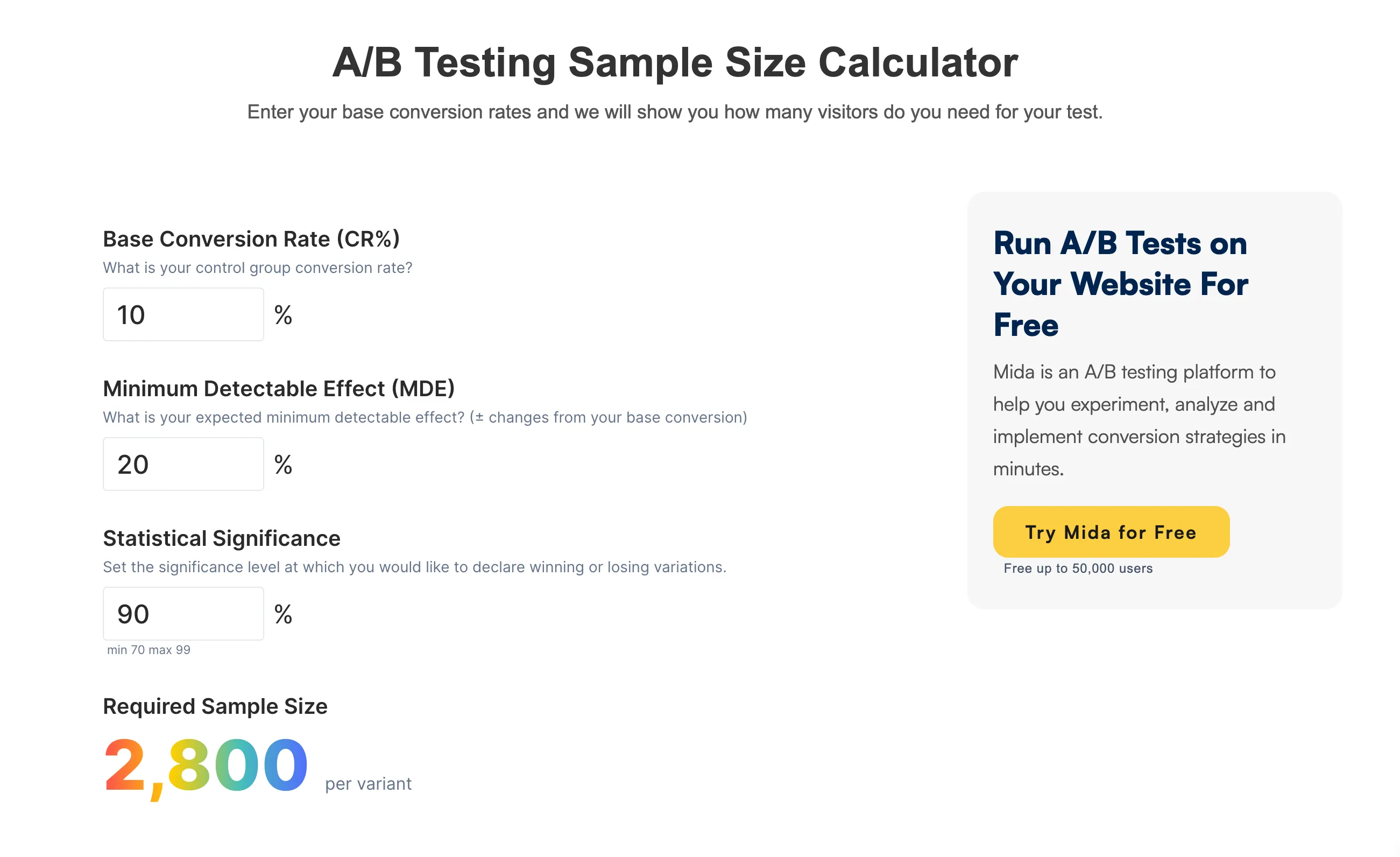
By determining these factors, you can calculate the optimal sample size needed for your test, ensuring you’re not stopping your test too early or running it when you’ve already reached a definitive conclusion.
Mistake 3: Ignoring Statistical Significance
When analyzing data from your A/B tests, it can be quite tempting to focus primarily on the conversion rates or other performance indicators. While they're undoubtedly important, they don't offer the complete picture without statistically significant evidence backing them up.
Put simply, statistical significance is a mathematical calculation that quantifies the probability that the difference in performance between the two variants was not due to chance. It tells us whether we can confidently attribute an observed change in conversion rates to the adjustments we made rather than random fluctuations in data.

Ignoring statistical significance can lead us down a problematic path, causing us to make decisions based on mere observations, devoid of any robust evidence characterizing their validity.
A closely related concept is confidence levels, and the industry standard is typically 95%. This means that if you were to repeat your experiment 100 times under the same conditions, you’d expect the same outcome 95 times. It's a way of measuring how certain we are that our A/B test results didn't occur by mere luck or chance.
But, what does that look like practically?
It means considering the p-value, a decimal between 0 and 1 that represents the likelihood of your results having happened randomly. Usually, a p-value of less than 0.05 (representing a 5% likelihood) matches a 95% confidence level, suggesting significant results.
The lower your p-value, the more certain you can be that the changes you made led to the differences you're seeing. A higher p-value (over 0.05) means there's a greater chance that the results you saw can be attributed to coincidence.
While 95% is the generally accepted threshold, the confidence level isn't a 'one-size-fits-all' scenario. If the changes being tested have significant potential impacts (positive or negative), you might decide to be more conservative and use a 99% confidence level.
Mistake 4: Cherry-Picking Results
When it comes to A/B testing, it's human nature to get excited at the prospect of success. It's not uncommon for marketers to breathe a sigh of relief and wrap up a test prematurely when initial data suggests their new webpage design or headline is outperforming the control variant. Unfortunately, this knee-jerk reaction can lead to skewed results and mistaken assumptions.
This approach to A/B testing is typically termed 'cherry-picking' results. It's the practice of prematurely ending a test and declaring a winner as soon as you start seeing positive results. It's as if in a 100-metre race, you decide the winner at the 20-metre mark just because one runner is ahead at that moment.

There's also a term for this; it's called 'Peeking'. Peeking at your results before the test has concluded substantially increases the risk of making changes based on false positives or negatives, which are misleading results that suggest a meaningful change when there has been none or vice versa.
To avoid the risks associated with cherry-picking and peeking, you must set clear testing protocols before commencing the A/B test. Determine the duration of your test and the minimum sample size in advance and stick to it. Even if initial results show strong favour towards a particular variant, resist the urge to declare a winner prematurely. Ensure the test runs its full course and reaches the desired sample size to maintain the integrity of the results.
This disciplined approach can be challenging to maintain, especially if early data points to a clear winner. But, it's crucial to remember that A/B testing is a marathon, not a sprint. Impatience and hasty decisions can lead to misinformed changes that may not bring about the intended improvements or could even adversely affect user experience.
Always remember the principle: 'Extraordinary claims require extraordinary evidence.'
If the new variant appears to be significantly outperforming your control, it becomes even more important to validate this finding with a comprehensive and robust A/B test that upholds established protocols. By doing so, you'll help shield your business from costly mistakes and pave the way for genuine uplifts in your site's performance.
Mistake 5: Overlooking External Factors
Your performance can be heavily influenced by a variety of external variables that have nothing to do with the changes you're testing. While you can't control all of these, you need to be mindful of them because they could significantly skew your results.
For example, suppose you're an e-commerce company conducting an A/B test during the holiday season. Sales usually spike during this period due to the increased consumer spending. If you make changes to your website at this time and notice an uptick in sales, it's easy to attribute this increase to your changes. However, it may just be the result of the seasonal shopping rush and have nothing to do with your modifications.
Here are some possible external factors you need to consider while analyzing your A/B test results:
- Seasonal Factors: These include holiday seasons, weekend and weekday variations, paydays, weather seasons and more. Each industry would have its own unique set of factors – for example, for the travel industry, it could be school vacation periods.
- Marketing Campaigns: Running significant marketing campaigns concurrently with your A/B tests could also impact the outcomes. These campaigns may bring atypical traffic to your site, which behaves differently from your regular audience.
- Site Outages or Crashes: Technical glitches could also hamper your results. Your data might be skewed if issues drive users away from your site or stop it from completing actions.
- News & Events: Industry news, regulatory changes, or significant societal events could also temporarily influence user behavior.
While it might not be feasible to control every factor, being aware and accounting for these external influences can go a long way in obtaining genuine, undistorted results from your A/B tests.
Consider designing your test environment to factor in regular patterns like daily or weekly trends. Or, you could conduct tests during a defined period where you don't expect significant external events or disruptions.
Furthermore, you might need more sophisticated statistical models like multi-arm bandit testing or setting up specific control groups if your A/B testing setup is intricate and large-scale and involves various external factors.
With a careful, methodical approach that considers the influence of external factors, you can yield more accurate and reliable insights from your A/B tests, empowering you to make evidence-based business decisions and achieve your website goals.
Conclusion
A/B testing can be a powerhouse methodology, but only when done correctly. Avoid these common pitfalls, and you will be well on your way to making data-driven decisions that boost your conversions and user experience.