Exploration vs Exploitation in A/B Testing

A/B testing in online marketing is a balancing act, a dance between the realms of exploration and exploitation. How do we find equilibrium in this dynamic? Let’s break it down.
What is Exploration and Exploitation?
Online marketing involves two fundamental approaches: exploration and exploitation.
Exploration
Exploration involves experimenting with new strategies, breaking away from the norm, and discovering potential pathways to success. It includes testing new advertising platforms, trying unconventional headlines, and implementing different website designs. It carries risks but provides valuable insights and learning for future gains.
Exploitation
Exploitation focuses on leveraging known and proven strategies that have worked well in the past. It maximizes existing resources and applies successful tactics repeatedly. For instance, replicating a high-performing ad campaign or email marketing strategy with minimal modifications. It is less risky and offers immediate rewards but can lead to stagnation without innovation.
Exploration and Exploitation in A/B Testing
A/B testing is a popular method for balancing exploration and exploitation. It involves comparing the performance of several versions of an ad, website, or other user experiences against each other to determine which one is the most successful. While A/B testing has its merits, it does have a few potential pitfalls.
One of the primary challenges of conventional A/B testing involves the distribution of traffic (or audience) between the different versions that are being tested. More often than not, traffic is split evenly between the versions. This leads to a 50/50 distribution of visitors between version A (generally the existing version or control) and version B (typically the new or experimental version).
Ideally, this equal distribution ensures a fair test, giving both versions an equal chance to exhibit their performance. However, problems arise when one version performs significantly better than the other.
The Risks

If version B starts outperforming version A, every visitor exposed to version A is essentially a lost opportunity. You could have had better metrics like higher click-through rates, more sign-ups, or more purchases if those visitors were presented with version B instead. This is an inherent flaw in traditional A/B testing known as "regret" - the loss experienced by not capitalizing on the best options.
Furthermore, conventional A/B testing requires a sufficient amount of time and traffic to reach statistical significance - in other words, to be sure that the results are not due to random chance. This can often mean that less effective versions may be running (and consuming resources) for longer periods.
Lastly, there is the issue of dynamism. The digital world is always in flux - customer behavior fluctuates, as do sales cycles, competitor actions, and even world events like the pandemic. The results of A/B tests are static and become less relevant over time, potentially making decisions based on these tests less reliable in a rapidly changing environment.
Now, all this doesn’t mean that A/B testing is not useful. It's still a valuable method of comparison, especially when used judiciously and in combination with other methodologies. However, being aware of its limitations helps to use it more effectively and maximize the potential of online marketing.
Multi-Armed Bandit
To address the challenge of traffic distribution in A/B testing, we have the Multi-Armed Bandit method. This solution adjusts the traffic allocation between various options based on their performance and potential, aiming to minimize regret (loss that comes from not choosing the optimal version) and maximize reward.
A/B Testing vs Multi-Armed Bandit
A/B Testing works best when the business doesn’t have a strong hypothesis about what will work, and there’s enough budget and time to run the test. On the other hand, the Multi-Armed Bandit approach is best suited when you need to quickly optimize experiences and can’t afford unnecessary exploration, or when the same test needs to be executed many times.
Tutorial: How to implement MAB?
Mida.so is an AI-powered A/B testing platform that businesses can use to automate A/B testing, freeing up resources to focus on other crucial areas.
Now, let’s go through the simple steps to set up a multi-arm bandit experiment on Mida.so. If you don't have an account yet, you can create a free account here.
Step 1: Create a new experiment
In the dashboard, click on Create a new test button, select A/B test to start a new experiment.

Step 2: Setup the experiment
Provide an experiment name, hypothesis, metrics, and other necessary details until you reach Configuration tab.

Step 3: Traffic Allocation
Here’s where the magic happens.
Instead of assigning traffic evenly, select the Automatically give more traffic to better-performing variants option. This enables machine learning to learn from the data gathered during the test to dynamically adjust visitor allocation in favor of better-performing variants.
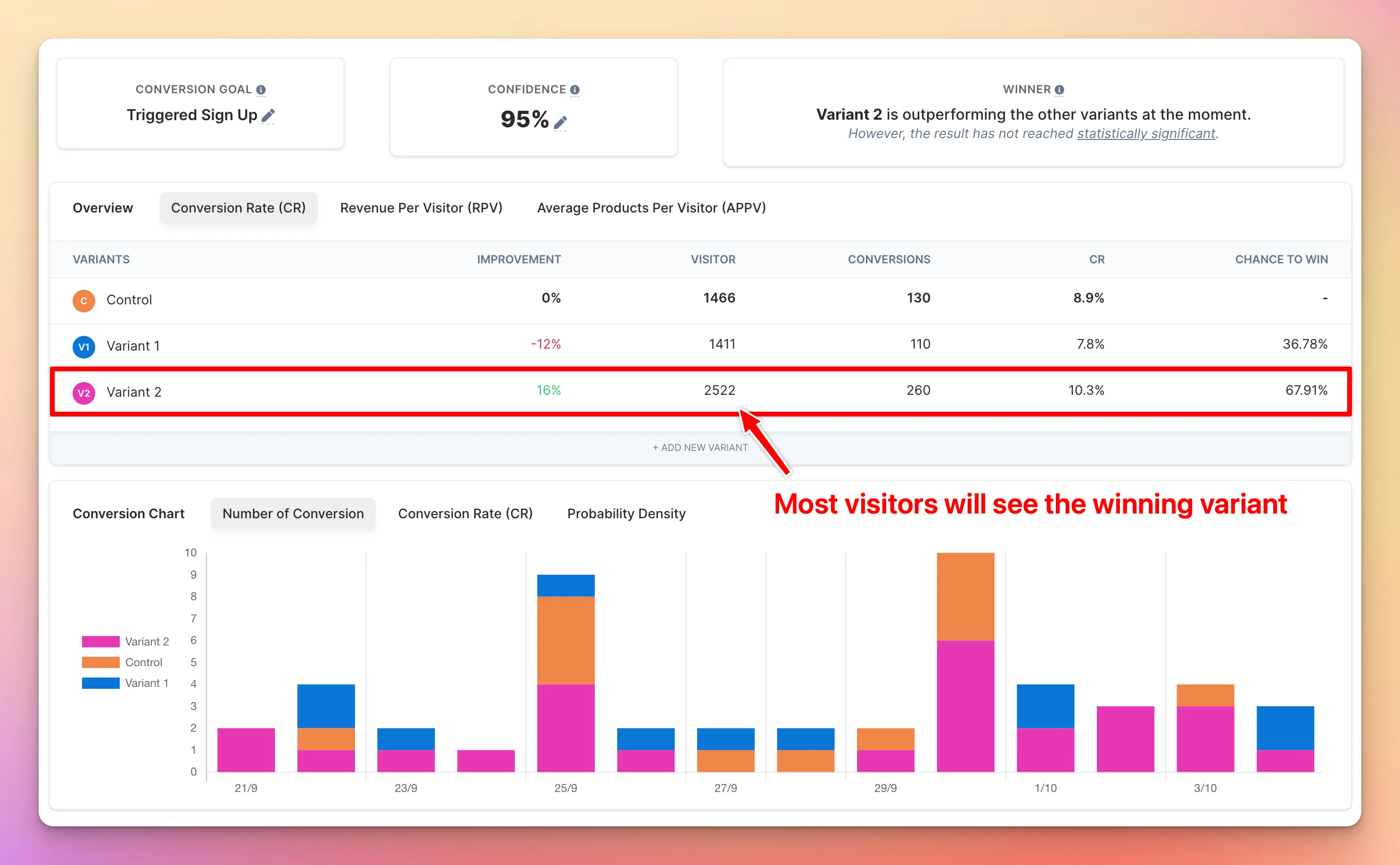
And voila! You’ve set up your Multi-Arm Bandit experiment using Mida.so. Balancing exploration and exploitation is crucial, and with the powerful combination of A/B testing and AI, Mida.so aims to offer a solution that is both effective and efficient. Enjoy the optimization journey!
Note: Mida offers a free plan up to 50k unique users. It’s a great way to explore the power of AI-powered automated A/B testing.
Remember, whether choosing A/B testing, the Multi-Arm Bandit, or a combination, the ultimate aim is to align your choices to your business goals and resources. Always test, learn, optimize, and repeat!